The Los Angeles Police Department’s Real-Time Analysis and Critical Response Division (RACR) is housed in a hulking, institutional-gray building about a mile north of downtown. Its only marking is its address: 500. In the fall of 2013, I had an early morning meeting there with Doug, a “forward-deployed” engineer from Palantir Technologies, which builds and operates one of the premier platforms for compiling and analyzing massive and disparate data used by law enforcement and intelligence agencies. He was one of about eighty people I interviewed over the course of five years to understand how the LAPD uses Palantir and big data.
Palantir’s goal is to create a single data environment, or “full data ecosystem,” that integrates hundreds of millions of data points into a single search. Before Palantir, officers and analysts conducted mostly one-off searches in siloed systems: one to look up a rap sheet, another to search a license plate, another to pull up field interview cards, and more still to search for traffic citations, access the gang system, and so on. Seeing the data all together in Palantir is its own kind of data.
Palantir’s clients—federal agencies such as the CIA, FBI, Immigration and Customs Enforcement, and the Department of Homeland Security; local law enforcement agencies such as the LAPD and New York Police Department; and commercial customers such as JPMorgan Chase—need training in order to learn how to use the platform, and they need a point person to answer their questions and challenges. That’s where engineers like Doug come in.
In a training room at the RACR, I watched as Doug logged in to Palantir Gotham, the company’s government intelligence platform, and pulled up the homepage (Figure 1). For him, it was a banal moment, but I’d been eagerly anticipating this sight: there is virtually no public research available on Palantir, and media portrayals are frustratingly vague.
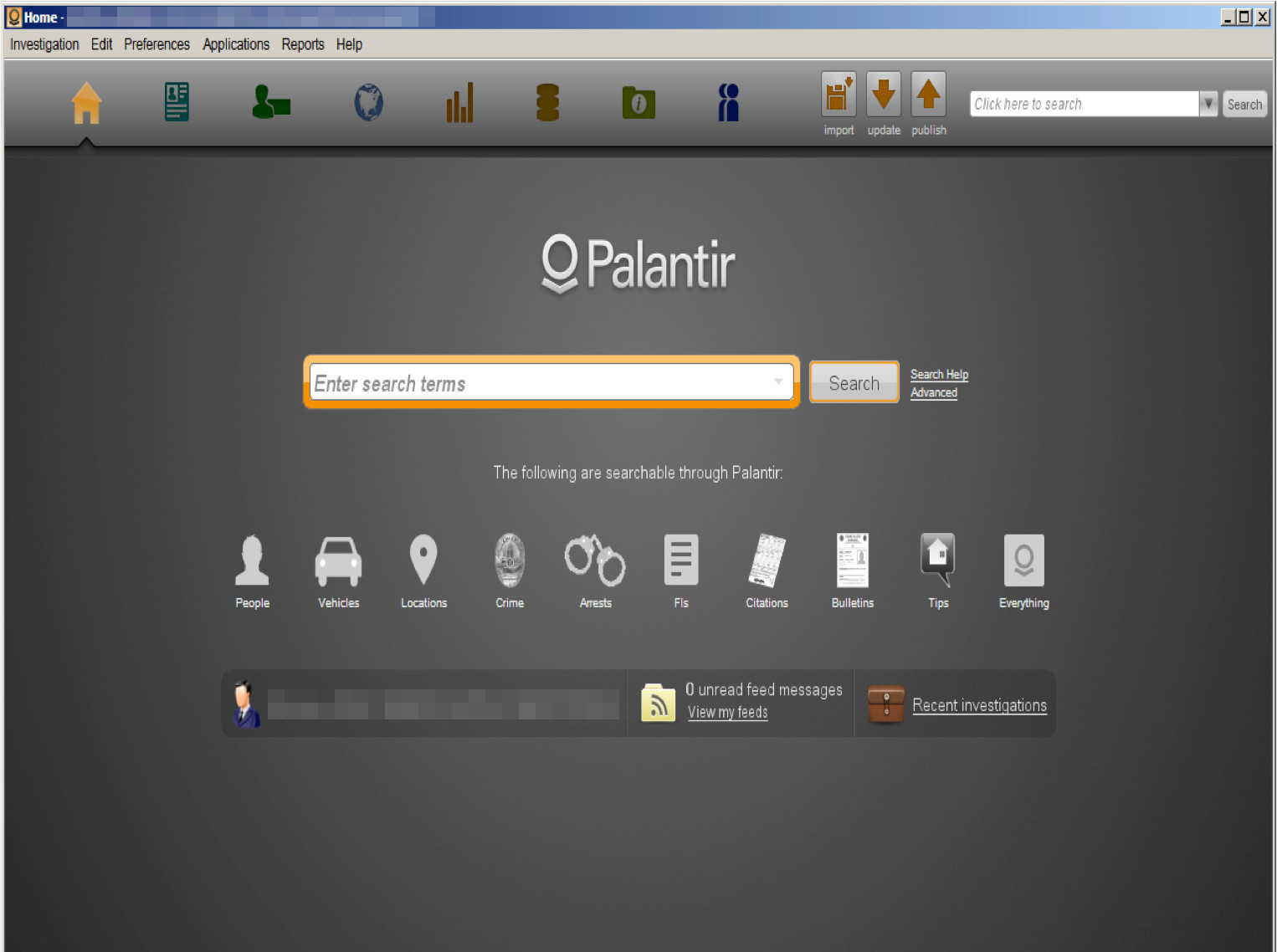
Doug started running through some of the different ways the platform could be used. He patiently explained what he was doing as he queried, clicked, zoomed, narrowed, and filtered:
So now, imagine a robbery detective who says, “Hey, you know what, I have a male, average build, black four-door sedan.” Like, they would [previously be able to] do nothing with that, right? So, we can do that.
Let’s go take a look at vehicles that are in the system… There are 140 million records in this system … we know it’s a Toyota, maybe a Hyundai, right? Or a Lexus… So let’s say we think it’s one of those types of vehicles, right? And that got us then to 2 million [vehicles]. And if we were to go look at, say, a color … we know it was black. Maybe it was blue, ’cause it could have been blue. It could be dark green… And we know it was a four-door.
Do you see what’s happening over here? In five hops, they’re able to get down to 160,000. Now they’re still not going to look at 160,000 vehicles. We didn’t get into model and year, but we could do that, and we could chart it, which makes it easy… So now I could say, I think it was between 2002 to 2005, drill down, now we’re 23,000. Now it gets pretty manageable.
So now let’s flip over and let’s look at the people that are connected to these vehicles. And I know I’m looking for a male. And I’ll just do one of them.
And I know that, like, let’s say he was pretty short. And he was on the heavier side. Brick house. We just got down to thirteen objects, thirteen people. And you could say, “Okay, well, now let me take a look at—all thirteen have driver’s license numbers.” So now we’ve narrowed it down to thirteen potential people, and they could take these thirteen objects and go to the DMV and pull their DMV photos and go to the witness or victim and say, “Here you go.”
In less than a minute, using partial information, Doug was able to narrow a search from 140 million records to thirteen. He went on to show me how to look up which of the thirteen had any citations or arrests, the LAPD divisions in which they received their citations or were arrested, and identify one person who had been cited in the same division in which the robbery occurred. If the person ended up not being the person who committed the robbery, officers could save this search formula and keep running it in the coming days, in case any new data came in.
I asked what happens when the system gives a false positive. What happens to the wrong suspect? Doug said bluntly, “I don’t know.”
One Person’s Click Is Palantir’s Clue
We all leave hundreds of digital traces—clues, should it come to that—every day. When we use our cell phone, run an internet search, or buy something with a credit card, we leave a digital trace. Rapidly proliferating automatic data-collection sensors record and save those digital traces, and make dragnet surveillance—the collection and analysis of information on everyone, rather than only people under suspicion—possible at an unprecedented scale. Individuals with no direct police contact are now included in law enforcement systems, and police now collect and use information gleaned from institutions, like credit-rating agencies, that are typically not associated with crime control.
But data—particularly large, diverse sets of data—are relatively useless on their own. You need a good platform to sort through them. And Palantir is excellent at processing, sorting, and analyzing data. With the right platform, searches that used to take hours, days, or even weeks may now take only a few seconds. Dragnet surveillance—and the data it produces—can be incredibly useful for law enforcement to solve crimes. As one officer explained, after any crime, “the first thing you’re gonna do, always, is check the digital footprint.”
People working in information technology have a vested interest in making the case that information technology is a crucial component of law enforcement. But no matter how you quantify it—through increased federal and within-department funding for data-intensive policing, the proliferation of law enforcement contracts with tech companies, the increase in tech-training sessions for police, or the rising number of data points the police access daily—data analytics are central to law enforcement operations today.
The Southern California fusion center Joint Regional Intelligence Center (JRIC), a multiagency, multidisciplinary surveillance organization, started using Palantir in 2009 to connect and analyze Suspicious Activity Reports (SARs). At the time, it was the largest law enforcement deployment of this software anywhere in the world. The LAPD, Long Beach Police Department, and LA City Fire Department soon adopted the platform, and there were over 1,300 trained Palantir users in the region by 2014. More users are onboarded every month. A sergeant named Michaels, who coordinates some of the training sessions at JRIC, claims “they catch bad guys during every training class.”
The LAPD’s arrest records and field interview cards—small, double-sided index cards that officers fill out with key information about people they interact with in the field—were the first data sources integrated into Palantir. Both are geocoded, meaning you can plot where these police stops and arrests occurred. Palantir does not own the data the LAPD uses, but rather provides an interface that makes it possible to link data points across previously separate systems. Users can plot data on maps, as a network, as a time wheel, or as a bar graph with a timeline of phone calls and financial transactions, for example. The platform even allows users to organize structured and unstructured data content such as emails, PDFs, and photos through tagging.
Because one of Palantir’s biggest selling points is the ease with which new, external data sources can be incorporated into the platform, its coverage grows every day. LAPD data, data collected by other government agencies, and external data, including privately collected data accessed through licensing agreements with data brokers, are among at least nineteen databases feeding Palantir at JRIC. The data come from a broad range of sources, including field interview cards, automatic license plate readings, a sex offender registry, county jail records (including phone calls, visitor logs, and cellblock movements), and foreclosure data.
Though there was a lot of uncertainty among my interviewees about exactly what data were in the databases they were accessing, a pair of civilian employees mentioned the use of LexisNexis’s public records database Accurint and speculated that it contained documents like utility bills and credit card information. Indeed, LexisNexis has over 84 billion public records from 10,000 diverse data sources, including 330 million unique cell phone numbers, 1.5 billion bankruptcy records, 77 million business contract records, 11.3 billion name and address combinations, 6.6 billion motor vehicle registrations, and 6.5 billion personal property records.
The process of labeling and linking objects and entities like persons, phone numbers, and documents makes it possible to plot data on maps and graphs that let users see data in context and make new connections. It can also make it easier to see what crucial data might be missing or what sorts of data might be useful for law enforcement to begin collecting. Whereas one piece of information may not be a useful source of intelligence on its own, Doug explained, the “sum of all information can build out what is needed.”
The Danger Imperative
Most sworn officers and civilian staffers, including crime analysts, who use Palantir Gotham use it for simple queries (what Palantir calls “drilling down using ‘object explorer’”). Users can search for anything from license plates to phone numbers to demographic characteristics, and a vast web of information will be returned. One officer described the process:
You could run an address in Palantir, and it’s going to give you all the events that took place at that address and everyone who’s associated to those events… So if it’s a knucklehead location where a lot of things are happening there, you’re gonna get people documented on there one way or the other… Either field interview cards, or they’re on crime reports, whatever… Otherwise you could search all of the records within [LexisNexis’s] Accurint … and see who’s living with who a lot of times.
It’s not quite so simple as “run a query, get a list of suspects.” Notice, for example, how the officer says “knucklehead location”—he means that you can query an address, and if it’s been listed as the address for many people or their car registrations, if it’s been the site of multiple calls for service, or it’s otherwise connected across the databases, a Palantir Gotham search is going to return a tangle of information. Some of it will be useful, some of it won’t, but the presumption is that if criminal activity is going on at a location, someone or something will be in Palantir.
Another employee at Palantir demonstrated how the platform can be used for retroactive investigative purposes: Law enforcement had a name of someone they thought was involved in trafficking. They ran a property search, which yielded the person of interest’s address and date of birth. Then they ran a search for common addresses (whether there are any other people in the system associated with the same address). One turned out to be a sibling of the initial person of interest, which sent investigators searching again, this time coming up with a police report for operating a vehicle without a license. They also searched the address of a third sibling, who lived at a different address. A radius search revealed several tips concerning this same house: one neighbor had called in to report a loud argument, and another reported that a suspicious number of cars was stopping at the house.
With this information, the police were able to set up in-person surveillance and subpoena phone records, which were run through Palantir’s “time wheel” function to identify temporal patterns. Modeling revealed phone calls to one or two phone numbers at the same time each week; using those phone numbers, police got a new database hit. They found a name and a police report and identified their suspect.
In another instance, I saw a user search for a car using just a partial license plate. They entered “67” and accessed all of the crime reports, traffic citations, field interview cards, automatic license plate readings, names, addresses, and border crossings associated with cars whose license plate contained these numbers in this order.
Advanced analytic tools on the platform include geo-temporal and topical analysis, each of which can be visualized differently. For example, users can plot (geo-analysis) all the types of crime they are interested in (topical analysis) during a given period of time (temporal analysis). Users can visualize the data on a map or along a chronological axis, as well as conduct secondary and tertiary analyses in which they analyze the results by, for example, modus operandi (e.g., using a bolt cutter) or proximity of robberies to a parolee’s residence.
Another way to use the analytic suite is to paint a detailed picture of the population of interest in an area. One officer explained this:
The big thing that Palantir offers is a mapping system. So, you could draw out a section of [his division] and say, “Okay, give me the parolees that live in this area that are known for stealing cars” or whatever [is] your problem… It’s going to map out that information for you … give you their employment data, what their conditions are, who they’re staying with, photos of their tattoos, and, of course, their mugshot. [And it will show] if that report has [a] sex offender or has a violent crime offender or has a gang offender. Some are in GPS, so they have the ankle bracelet, and … we have a separate GPS tracker for that.
A Palantir software engineer spoke of the gang unit monitoring entire networks of people: “Huge, huge network. They’re going to maintain this whole entire network and all the information about it within Palantir.”
Palantir, one sergeant explained, is also an “operational game changer”: it gives him the data he needs to protect his officers’ safety by, for instance, locking down a neighborhood and positioning an airship overhead while law enforcement conducts a search. Of course, this situational awareness made possible by Palantir can ratchet up officers’ sense of danger and escalate an already tense situation. Such platforms provide an unprecedented number of data points supporting the “danger imperative,” or the cultural frame officers are socialized into, which encourages them to believe that they may face lethal violence at a moment’s notice.
Criminal Justice Creep
New data sources are incorporated into Palantir regularly. One captain commented:
I’m so happy with how big Palantir got… I mean it’s just every time I see the entry screen where you log on there’s another icon about another database that’s been added … they just went out and found some public data on foreclosures, dragged it in, and now they’re mapping it where it would be relative to our crime data and stuff.
Another interagency data integration effort is LA County’s Enterprise Master Person Index (LA EMPI) initiative. If established, LA EMPI would create a single view of an individual across all government systems and agencies: all of their interactions with law enforcement, social services, health services, mental health services, and child and family services would be in one place under a single unique ID. Although the explicit motivation behind the EMPI initiative is to improve service delivery, such initiatives extend the governance and social control capacities of the criminal justice system into other institutions.
This is one of the most transformative features of the big data landscape: the creep of criminal justice surveillance into other, non–criminal justice institutions. I encountered many examples of law enforcement using external data originally collected for non–criminal justice purposes, including LexisNexis, but also TransUnion’s TLOxp (which contains one hundred billion public and proprietary data points, including social security numbers, employment records, and address records); databases for repossession and collection agencies; social media, foreclosure, and electronic toll pass data; and address and usage information from utility bills.
Respondents added that they were working on integrating hospital, pay-parking lot, and university camera feeds, as well as rebate data, pizza chain customer lists, and so on. One interviewee in the LAPD’s Information Technology Division said they had their eye on consumer data: “Other stuff, shopping data. You can buy it, you know, certainly other vendors are. So why not?” In some instances, it is simply easier for law enforcement to purchase privately collected data than to rely on in-house data, partly because there are fewer protections and less oversight over private sector surveillance and data collection.
Another of the most substantively important shifts that have accompanied the rise of big data policing is the shift from query-based systems to alert-based systems. By “query-based systems,” I mean those databases that operate in response to a user query, such as when an officer runs your license plate during a traffic stop. In alert-based systems, by contrast, users receive real-time notifications when certain variables or configurations of variables become present in the data. High-frequency data collection makes alert-based systems possible, and that carries enormous implications for the relational structure of surveillance.
Imagine an officer wants to know about any warrants issued for residents of a specific neighborhood. In a query-based system, they would need to set up specific searches, and most of those would be useful only well after the warrant had been issued. All of the millions of warrants in LA county are geocoded and can be translated into object representations spatially, temporally, and topically in Palantir. Through tagging, users can add every known association of a warrant to people, vehicles, addresses, phone numbers, documents, incidents, citations, calls for service, automatic license plate readings, field interviews, and the like. All that information is cross-referenced in Palantir. Then, using a mechanism in Palantir that’s similar to an RSS feed, an officer can set up automatic notifications for warrants or events involving specific individuals (or even descriptions of individuals), addresses, or cars to ping their cell phone.
For example, an alert can be set up by putting a geofence around a given area and requesting an alert every time a new warrant is issued within that perimeter. One sergeant had an email alert set up in this way, and could even get the alert while he was out on patrol. “Court-issued warrant, ding!” As soon as he got the notification, he says, he was able to track down and arrest the suspect. Previously, the process was far slower. “Now,” he explained excitedly, “you draw a box in Palantir and go about your business. Ding!”
A civilian employee described a similar approach using automated license plate readings: “If you have an automated license reader, you can flag a plate or a partial plate and you could attach it to your email. And if it ever comes up, it will send you an email saying, ‘Hey, this partial plate or this vehicle, there was a hit last night. Here is the information.’”
Becoming Carmen Sandiego
Law enforcement databases have long recorded who has been arrested or convicted of crimes. Today, they also include information on people who have been stopped, as evidenced by the proliferation of stop-and-frisk databases. The real surprise may be that as new data sensors and analytic platforms are incorporated into law enforcement operations, the police increasingly utilize data on individuals who have not had any police contact at all.
The automatic license plate reader (ALPR) is perhaps the clearest example of a low-threshold “trigger mechanism,” lowering the bar for criteria that justifies inclusion in databases. ALPRs are quintessential dragnet surveillance tools—they take readings on everyone, not just people under suspicion. Their data come in the form of two photos—one of the license plate and one of the car, along with the time, date, and geo-coordinates attached to those photos, as read by ALPR cameras mounted on the tops of police cars and static cameras at intersections and other locations. ALPR data collected by law enforcement can be supplemented with privately collected ALPRs, such as those used by repossession agents. Just this one relatively simple technological tool makes everyday mass surveillance possible on an almost unimaginable scale.
In addition to ALPRs, there are all sorts of low-threshold trigger mechanisms being leveraged by the LAPD. Much of the data is what’s being called “collateral data collection,” and it is a passive, pervasive way people are being caught up in the surveillance state. Figure 2 is a de-identified notional representation, based on a real network diagram I obtained from the LAPD.

The Carmen Sandiego–looking figure in the middle, “Stephen Thompson,” is a person with direct police contact. Radiating outward, we see all the entities he is related to, including people, cars, addresses, and cell phones. Each line indicates the type of connection (e.g., sibling, lover, co-arrestee, vehicle registrant).
To be in what I call the “secondary surveillance network,” radiating out from the person of interest, individuals do not need to have direct law enforcement contact; they simply need a connection to the central person of interest. And once they are in this system, these individuals can be autotracked, meaning officers can receive real-time alerts should they come into contact with the police or other government agencies.
When many streams of information flow together, they form a “data double,” which can be a powerful tool in the hands of law enforcement. As a member of legal counsel at Palantir explained, digital traces can be knit together so that circumstantial evidence looks like a comprehensive picture: there is “usually not one smoking gun document, but we’re able to build up a sequence of events prosecutors might not previously have been able to do … [we can integrate] data in a single ontology to rapidly connect illicit actors and depict a coherent scheme.” This reconstruction may be invisible to civilians—and to their lawyers, if they end up being charged.
But indiscriminate data collection is not the inevitable outcome of technological advancement. Mass surveillance is not the “natural” result of mass digitization. Instead, what we allow to proliferate and become the objects of massive data-collection efforts are choices that reflect the social and political positions of the subjects and subject matter that we feel comfortable surveilling.
As a counterpoint, consider guns in the United States: we do not permit the mass tracking of guns. There is no federal gun registry, and the National Instant Criminal Background Check System is required by law to destroy the audit logs of background checks that go through its system within ninety days. We certainly have the technology to track guns, and we could easily leverage existing technology to do more tracking, but gun owners are powerful political subjects. They have the resources to assert that their guns should not be tracked.
Police officers, too, have routinely invoked their authority and legitimacy to undermine attempts to surveil their work lives. They have the power to resist in ways that their more usual subjects, disproportionately low-income, minority folks with little political capital and no small amount of fear, cannot. In that way, too, dragnet surveillance serves to reinscribe inequality.